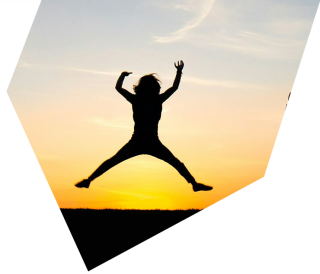
There is no shortage of opportunities for artificial intelligence (AI) and machine learning (ML). Still, we need to stop thinking of them only as tools for automation, operational uplifts and efficiency gain.
There are opportunities that extend beyond the day-to-day, explore new ground, and open up areas that are not obvious, yet deliver significant value and benefit. These opportunities can be found in almost every industry, and by way of example, there have been exciting advances in AI and ML supporting mental health in the military.
For several years, health professionals and technologists have been working on advances in medical technology that use AI and ML, particularly in health conditions that are more prevalent in the military. These advances have often taken innovative approaches to preventative care and medical diagnosis, and looked for ways to be more sensitive and less intrusive to soldiers already facing extreme hardship.
A great example is the use of AI and ML in identifying post-traumatic stress disorder (PTSD) in defence force members. PTSD is the second most common mental health condition after depression. A study by the Department of Veterans’ Affairs (DVA) in 2018 found that the 12-month prevalence rate of PTSD was 8% for currently serving members of the ADF and 17.7% for members who have transitioned from full-time service. A PTSD diagnosis is complex, with the onset of symptoms often coming sometime after the trauma. The subsequent care and support provided is a journey that can take many paths, and AI and ML’s current role is helping diagnose PTSD so that the journey can begin.
Much like monotone speech is an indicator of depression, researchers have studied whether PTSD indicators might manifest in speech patterns. In a study published in the US in April 2019, AI software was used to identify 40,526 different characteristics in speech that could be used to classify PTSD accurately in 89.1% of cases. This meant that AI was able to provide a very accurate diagnostic alternative that was objective, inexpensive and less intrusive than the commonly used diagnostic questionnaires.
Another study published in September 2019 leveraged AI to find biomarkers in the bloodstream, the first test of its kind for a psychiatric condition. The research used AI to study subtle changes in biomarkers, identifying 27 characteristics that could identify PTSD with 85% accuracy.
More recently, a study published in July 2023 leveraged AI to predict PTSD in a military population before deployment. The researchers looked at soldiers one to two months before deployment and then again three to nine months following return from deployment. The assessment considered 801 potential indicators, and one-third of those identified as high-risk soldiers accounted for 62.4% of PTSD cases, proving the model to be a good indicator for PTSD risk and, therefore, early intervention.
Although these studies and findings are in their early stages, they demonstrate the potential of AI to identify, assess, and analyse large volumes of complex data to derive real insights quickly and accurately.
Besides PTSD, a significant number of ADF veterans self-report heart conditions. In late 2022, the use of AI in assessing and diagnosing heart function was more accurate than the echocardiogram assessments made by clinicians. This study gave cardiologists more accurate reports, and the process was quickly integrated into the clinic’s workflow. Ironically, the study intended to demonstrate that clinicians were more effective than AI, but AI made almost 10% fewer errors. The trials will continue, and embedding AI into heart care will also show benefits for ADF.
The work cited here would have traditionally required researchers to spend thousands of hours analysing a vast amount of data. With the maturation of AI and ML, they can use these technologies to spend more time on the real problem instead of crunching the numbers.
We need to open our minds to augment what we know with the computer processing available to improve decisions, outcomes, and experiences. Although we focused on the application of AI and ML in the highly complex area of medical diagnosis, its broader application is also ready for exploration.
The problem could be different within your organisation — how do you help your customers, build resilience into organisations, understand the risks, or provide better experiences — but the fundamentals are the same. If you organise your data well, you can explore the possibilities of AI or ML in a proof of concept or pilot to test the value first. Think about how this can be worked into your current workflows (it may be easier than you think) and what opportunities you might gain from patterns in your data that you had not noticed.