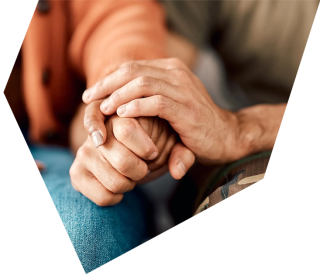
The increasing availability of compute power and vast data holdings has allowed generative AI to evolve from simple, deterministic algorithms to complex probabilistic models. It has quickly demonstrated exciting human-like abilities to understand natural language queries, respond with helpful data analysis, and generate entirely new audio-visual content, creating opportunities for the government to reshape how they deliver high-quality services safely and personalise customer experiences.
Like all statistical and machine learning techniques, the efficacy of generative AI is dependent on the volume and quality of data fed into it.
There is an inherent tension in training a generative AI model to be as valuable as possible while navigating the ethical and legal constraints that apply to the data it needs. Publicly available models trained using large quantities of freely available data, including copyrighted artistic works, sensitive personal information, and illegal material, have exposed organisations using them to financial and reputational damage and legal sanction where this data has surfaced in their outputs.
Generative AI models will also present their output as valid regardless of whether its training data is accurate, incomplete or biased. In some cases, AI “Hallucinations” are outputs when the model perceives patterns in data that are nonsensical to human observers. In other cases, generative AI has been used to deliberately create plausible text, images and audio that manipulate truth in media, politics and advertising.
Understanding training data defines the scope of what is possible. Identifying suitable training data requires considering the classic data quality dimensions, including accuracy, bias, and completeness, and constraints on its use within the organisational legislative, policy, and regulatory environment. Organisations should exhaustively test candidate training data with the organisation’s governing and regulatory groups, which is particularly important when a training model requires publicly available or purchased data.
Generative AI models also require new data and feedback. Otherwise, they become less accurate over time, and the risk of inaccurate and misleading output increases, raising additional risk of its use in the organisation. AI leaders should plan for the need to continually measure a generative AI model’s accuracy and suitability and continue the training over time.
Generative AI’s probabilistic nature and inherent lack of transparency make it difficult to determine how it arrives at its output or explain its reasoning. Decision makers and those staff incorporating generative AI into their day-to-day must understand these limitations and become AI literate.
Failure to successfully navigate the Generative AI hype cycle can lead to damaged reputation, negative social impact or even legal action. AI Leaders must ensure that potential generative AI use cases are anchored to specific business needs and aligned with its capabilities.
The strength of generative AI is in practical applications that significantly augment workflow processes. These use cases, often found in operations, service delivery and compliance, save time and drive cost savings by freeing staff for higher-value creative work and quickly inform the complex decisions organisations must make.
Case management is inherently a business function executed by knowledge workers, skilled experts who use their experience and knowledge to work with processes and policies to deliver a service that could have severe negative consequences if not done with sound human judgment.
Process workflow steps, as supported by case management, are discrete and modular, which lends themselves to integration with generative AI, which produces outputs that can be generated and attached to the case under human oversight. These outputs can be used then to augment the human understanding of the case and related decision-making and help tailor their interactions to the specific needs of their clients and stakeholders.
Possible applications within case management include:
These use cases require the considered use of governance and management of data used in training a generative AI model and its output. They still need mature process design and cultural change elements to be truly effective.
The rapid evolution of generative AI is outpacing existing legal frameworks, complicating AI’s governance and ethical use. Government AI Leaders should maintain a clear view of the use of generative AI in their organisation and its place in their organisation’s legislative and policy environment to remain agile and responsive as new guidance emerges.
Generative AI is a powerful tool. It can save time, ideate novel problem approaches and shortcut laborious data insight processes. However, AI leaders must consider its place in their data and analytics ecosystem and the broad set of risks surrounding its use.